Revolutionizing the role of AI in networking
This post was sponsored by AT&T Business, but the opinions are my own and don’t necessarily represent positions or strategies of AT&T Business.
Artificial intelligence (AI) has arguably taken the number one spot in the minds of business leaders worldwide leaving many to be stricken with AI FOMO (Fear of Missing Out). They’re overwhelmed by AI’s seemingly unending advantages and where to begin to implement it into their business. If this is you, you’re not alone.
Let’s start with the basics. There are two models for AI: traditional AI and generative AI. Traditional AI specializes in pattern recognition, data analysis, and making predictions. Examples include search and recommendation engines like Google or Amazon. Generative AI is what most people think of when AI comes to mind. It includes applications like Open AI’s Chat GPT, graphic design creation, and fraud detection.
And then there’s operational benefits such as how AI in networking can help you to mitigate your security risks.
What is artificial intelligence (AI) in networking?
Although many people still associate AI with science-fiction movies and futurist predictions, that characterization does not reflect its use in the modern business world. AI has rapidly developed in business and has become commonplace in customer interaction and support. While accepting AI in mainstream society is relatively new, it is not a new concept. Modern AI came into existence in 1956, but it took decades of work for it to progress and develop into today's AI business systems. This extension to business has made some of those sci-fi visions of AI into a reality.
Artificial intelligence is a field of study that gives computers human-like intelligence when performing tasks. When applied to complex IT operations, AI assists with making better, faster decisions, which enables business network process automation. Machine learning (ML) is one of the most common types of AI and represents its most common business use case. ML is primarily used to process large amounts of data quickly. These types of AIs are algorithms and can be used over time to mimic the human learning process.
Feeding data—purpose-specific quality data—into a machine-learning algorithm can help improve its ability to mimic a human response over time. This capability makes ML useful for putting vast troves of data—increasingly captured by connected devices and the Internet of Things (IoT)—into human digestible context.
Since AI uses machine learning, it can learn continuously by searching a global database with complex algorithms and deep learning (DL), which is a subset of machine learning. Deep learning simulates how humans think to perform tasks with increasing accuracy as it learns. It quickly finds anomalies and generates real-time insights. With this information AI can then use machine reasoning by applying the human reasoning it has collected to discover and propose new solutions.
Artificial intelligence can be used in many ways in business. We may not realize it, but most of us interact with AI every day through chatbots, facial recognition on your smart device, and personal digital assistants like Siri on Mac devices and Alexa on Android devices. And in business, AI is disrupting almost every process in every industry. As AI technologies proliferate, they’ve become critical to efficient business operations and to staying ahead of the competition.
In order to take advantage of the promises that AI offers, it must first understand the context of your business or your local network. How does AI do this? It uses the algorithms and data parsing (converting data from one format to another) of machine learning to learn about your local networking environment and specific business goals. This allows AI to make operational determinations or predictions without the need for a human to provide explicit instructions.
There has been a lot of advancements in computation and storage, which are driving the evolution of AI and ML. It’s creating more complex structured models like deep learning and neural networks. Natural language processing (NLP)—how programs interpret spoken and written human language—has also reduced the complexity of using these tools by using vocal and word-based recognition to improve computer interfacing. This blend enables for more timely, useful, and actionable operational insights and automation.
Network operations and functions in an AI environment
From a practical business point of view, many organizations have serious concerns about security planning and operations across their business ecosystem network. Work-from-home and pop-up network sites make threat-aware network implementations essential. AI can identify and physically locate compromised devices and appropriately react to related cyber threats when used in cybersecurity.
AI can supplement layered security, which begins with having a reliable, secure network. Combined with managed cybersecurity solutions, AI can help IT managers stay ahead of the rapidly evolving threat landscape. And because this is such an enormous undertaking, it’s imperative that they consult with third-party experts who can provide an objective risk analysis and roadmap the best approach to your company’s unique security needs.
In addition, IT teams can protect the networks and devices they don't directly control through risk profiling, even when business operations require connection to the network. AI can also enhance cyber defense by providing deep network visibility and enabling policy enforcement at every connection point. It also augments constant monitoring of applications and user connections across the network environment with behavioral context. This design automates a quick decision on whether the network use is acceptable, potentially anomalous, or malicious.
AI and machine learning can improve network automation even in an environment characterized by flat or shrinking IT budgets. One approach is to implement a virtual network assistant. This option provides predictive recommendations on how to avoid problems by combining quality data, domain expertise, and syntax (metrics, classifiers, root causes, correlations, and ranking). This AI solution reduces costs and offers actionable insights on remediating existing issues. Customer experience is simultaneously enhanced by automating learning wireless network nuances and automating the response to users' questions such as, "What went wrong?" and "Why did that happen?"
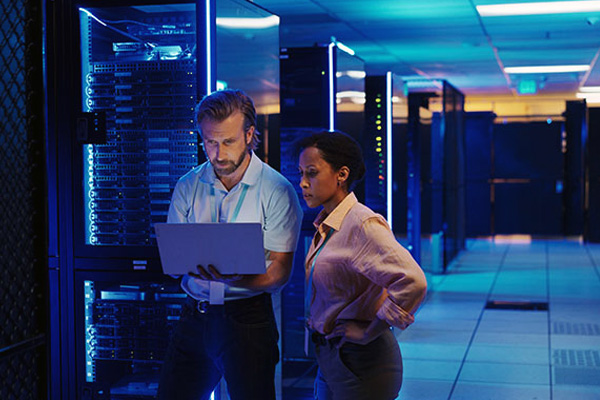
Using AI to drive business success
Using AI can be a game changer for scaling your business. Andy Markus, CDO from AT&T Business, discusses using AI for smarter business decisions.
How can we implement AI in networking?
A proven process for leveraging AI in networking to improve business performance, efficiency, and customer experience is adopting a two-phase implementation approach focused on the desired business outcomes and the data needed to measure goal attainment.
The overall strategy is to start small with an initial investment in a project suitable to your situation. The implementation should grow gradually from that first, well-designed, and established function while keeping the future in mind. Reuse different solution elements as the path forward becomes evident. By using this approach, your team will build confidence as it gains more flexibility and end-to-end experience in how to train the AI model, retrain, update, and deploy new capabilities.
This process starts by cataloging relevant end-to-end systems and processes in the business area targeted for automation. Next:
Phase 1: This phase centers on offloading low-level, repetitive, and low-risk tasks to free up humans to do more high-level work. It typically starts with the implementation of "standard" rule-based functionality. In this step, human experts manually create the rules by which automation frameworks operate. AI is used in a supporting role to inform the human-made decision-making process with predictions based on ML algorithms.
Phase 2: In phase 2, network AI systems shift from just another tool to becoming a virtual co-worker. Artificial Intelligence automates entire processes based on parameters, including making critical decisions. This step places much higher demands on AI regarding robustness, assurance, and recovery actions and will require reinforcement learning (RL). The culmination of this phase is a system that is fully AI-controlled. Humans only need to provide the high-level intent for the operation, like a Service Level Agreement, which includes the business-related requirements such as cost or priorities.
Can your network handle AI?
While concern about implementing AI on your network is warranted, viewing this step as a critical investment into your business's future is the right approach. This technology's new advances and use cases can offer better and more accurate business information. This capability helps enhance communications, automate routine tasks, and provide detailed insights into logistical operations, resulting in improved performance, increased customer satisfaction, and reduced operational costs.
The primary challenge to AI adoption lies in leadership's ability to separate hype from the essential insights on applying AI and ML to critical business processes. The expanded use of AI technology is changing how networks are set up, managed, and optimized. Approaches like AI Operations (AIOps) are moving networking from managing network elements to managing network user experience. AI is network automation transformation because it helps organizations build network management and operations solutions on par with human IT domain experts.
Beyond AI in networking: related technologies
Leveraging AI in your local network, however, is only the beginning. Modern operations across a globally networked environment also drive the need for IoT services, cloud connectivity, and a wide area networking security partner capable of delivering AI in networking value. If your enterprise implements AI in your local network, consider engaging AT&T 5G for Business as your wide area networking security partner. Collaborating with AT&T Business brings data and insights learned by operating the AT&T network, which includes being the nation’s largest fiber internet provider.1
Since any meaningful AI solution begins with massive amounts of quality data, AT&T Business continually uses data collection and analysis to build its network intelligence. AT&T also leverages the industry domain-specific innovations that formed the foundation of how the company designs, plans, and operates one of the world's largest communications networks.
The resulting metadata helps break network challenges into small segments used to train AI models. After dividing this data into domain-specific metadata, they leverage ML, big data analytics, and neural networks to provide actionable insights from that analyzed data. Insights that are gleaned are then used to power virtual network assistants that apply collaborative filtering to identify, correlate, and assemble an AI-informed solution to a specific customer problem.
Working with AT&T Business will help you deliver an optimized user experience to your customers while efficiently releasing your human capital to do other, higher-level work. Visit AT&T Business to learn more.
Learn more about AT&T Business solutions at business.att.com. And to connect with an expert who knows business and can help advise you how to set your foundation for AI adoption, contact your AT&T Business representative.
Why AT&T Business?
See how ultra-fast, reliable fiber and 5G connectivity protected by built-in security give you a new level of confidence in the possibilities of your network. Let our experts work with you to solve your challenges and accelerate outcomes. Your business deserves the AT&T Business difference—a new standard for networking.
1Based on network availability.